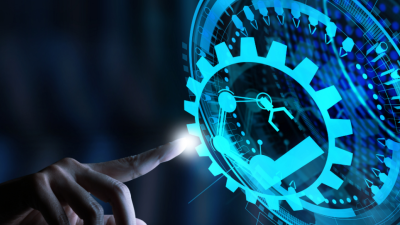
Reflections from The Alan Turing Institute’s AI UK
Reflections from The Alan Turing Institute’s AI UK.
This page is approximately a 2 minute read
This page was published on
The Lloyd’s Register Foundation-funded Assuring Autonomy International Programme (AAIP) at the University of York have published their latest guidance to support system developers, safety engineers, and assessors to design and introduce safe autonomous systems.
The new research, 'Safety Assurance of autonomous systems in Complex Environments (SACE)', marks an important milestone in providing a framework for the regulation and adoption of robotics and autonomous systems across a variety of sectors, ensuring these kinds of technologies can help contribute to a safer world in the future.
An autonomous system, such as a self-driving car or clinical support tool, does not operate in isolation: it is part of a complex environment. An autonomous vehicle will interact with other cars, traffic lights and street infrastructure, as well as with humans. An AI tool in healthcare will become part of a complex healthcare pathway that could involve numerous clinical staff and care options.
SACE takes the autonomous system and its environment, and defines a safety process that leads to the creation of a safety case for the system.
Dr Richard Hawkins, Senior Research Fellow at the Assuring Autonomy International Programme and one of the authors of the guidance, said: “The autonomous system, its environment, and the interactions that take place between actors in the environment must all be part of the assurance process, in order to demonstrate that the system is safe.
“Our Safety Assurance of autonomous systems in Complex Environments (SACE) guidance enables engineers and assessors to take a holistic view of the system within its environment. They can follow the activities in the eight stages that make up the SACE process. This will lead to the creation of artefacts that can be combined to create a compelling safety case for the system.”
This SACE guidance sits above AAIP’s previously published AMLAS guidance, the leading methodology for assuring the safety of machine learning components within an autonomous system.
“Our new system-level guidance sits above the AMLAS methodology,” continued Dr Hawkins. “The ML safety case created by following AMLAS fits into the system safety case that you build by following our SACE guidance. The two parts work together to help create a coherent and compelling safety case for an autonomous system.”
For more information and to download the SACE guidance, please visit the AAIP website.