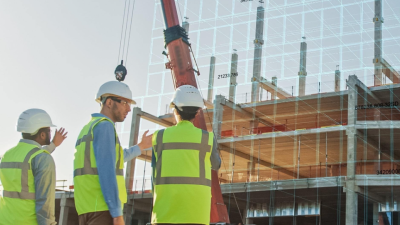
New technologies to improve health and safety performance
Discovering Safety joins forces with Safetytech Accelerator to build a Smarter Regulation Sandbox.
This page is approximately a 7 minute read
This page was published on
Dr Philippa Ryan, Senior Research Fellow
Philippa is a Lloyd’s Register Foundation Senior Research Fellow at the Centre for Assuring Autonomy, a partnership between the University of York and Lloyd’s Register Foundation.
Dr Philippa Ryan is a Lloyd’s Register Foundation Senior Research Fellow at the Centre for Assuring Autonomy, a partnership between the University of York and Lloyd’s Register Foundation dedicated to pioneering research and innovation at the intersection of AI and safety. In this article, Philippa reflects on how her fellowship allows her to explore different safety applications, and the ever-important and evolving relationship between the safety world and the AI landscape.
My first job as a graduate was at Leeds General Infirmary researching how to compress digital mammography images to save storage space while making sure that all the diagnostic information remained. The tension between a very practical issue (a terabyte drive took up a whole room back then!) and patient safety really fascinated me ever since. Safety is always a balancing act - and can be a real challenge.
After that, I came to the University of York to do my PhD in avionics software safety and was a research associate for a number of years before moving to the safety critical systems industry. Whilst working in industry as a software and system safety assessor and consultant, suddenly AI and autonomous systems became really big news. As a sci-fi nerd, AI has always interested me - but adding it to safety critical systems had been counter to good industrial practice. Most AI (especially machine learning) is really hard to assure and design safely. This was a deep research challenge I wanted to be involved in trying to solve. The work with the AAIP (now the Centre for Assuring Autonomy) at York really chimed with my views on good safety practices and current challenges, and I knew that researchers in the Programme are top of their field and part of the reason our research is industry applicable. This is the reason I decided to rejoin academia.
Working as an auditor and safety assessor gave me a real appreciation of scale. I worked on very varied systems from industries including aerospace, medical devices, maritime, and autonomous cars. The amount of documentation and effort needed to assure safety of even low risk systems can be huge and must be managed in a sensible way. Pragmatism is key to understanding strengths and weaknesses of the evidence, and you need to draw out the issues that really matter to the safety of the system. That’s something I try to keep in mind: any new methods I develop must be pragmatic, scalable and support focus on the key practical problems for safe deployment. This is in keeping with the ethos of the Centre, and what it is known for: having research that is applicable to industry.
Recently I worked on an interdisciplinary project, Assuring Responsibility for Trustworthy Autonomous Systems (AR-TAS), that spanned law, philosophy and computer science. This project really challenged my thinking on the moral and responsibility questions associated with autonomous systems and AI. For example, who would be legally responsible following an accident involving a fully autonomous system and what are the ethical questions relating to the introduction of AI in healthcare, reducing contact time with clinicians?
This is something I also explored in some of my recent work where I look at the problem of responsibility within the case of the autonomous vehicle crash in Tempe, Arizona, that occurred in 2018. The incident resulted in the death of a pedestrian and the prosecution of the safety driver, and it raises questions around the importance of role, moral, legal and causal responsibility when using AI and ensuring its safety. I think it’s really important not to forget the damage that these systems can do, and remember the human and environmental cost of getting things wrong. We can’t assume a human can react in time to a fault in AI, as AI fails in unpredictable and complex ways, but should design safety into the system from the start.
Over the last few years, AI has been introduced and is evolving at an incredible pace and it's appearing in many different systems and scenarios. The safety world has historically been slow and conservative, and this has led to a clash of cultures. Regulators are struggling to keep up with the innovation. As an example, recent research is showing that generated AI trained on its own output can suffer catastrophic problems not detectable by the usual metrics. Synthetic data is generated to create the difficult and rare situations in safety cases that can lead to a host of unforeseen challenges. One of my colleagues co-authored a paper which explores this topic.
Another change is in the types of harm people are focussing on. The safety community has tended to concentrate on physical risk, but the landscape is shifting to consider issues like bias against underprivileged groups and even existential risk. AI must be trained and used in a responsible and safe way to prevent this, as discussed in our recent research on Type II diabetes care. This is really challenging because it affects the boundaries of our work: changing the requirements for safe operation to consider, for example, long term impacts on underprivileged groups or on mental health. I think it’s important to not to lose sight of the immediate problems we face from AI though (such as bias or mis-diagnosis in healthcare, or accidents with autonomous vehicles), rather than focus too much on the sci-fi Matrix scenario of pervasive AI.
I’m very fortunate to have the support of Lloyd’s Register Foundation with a long-term research contract, allowing me to explore many different safety applications to further enhance the work of the CfAA. Recently I have worked with colleagues on a diverse range of projects from inspection drones to maritime autonomy, and clinical decision support to autonomous vehicles. At the core of these are common themes around responsible use of technology and the difficulty of assuring machine learning (ML) components. These support and align with Lloyd’s Register Foundation’s mission to engineer a safer world. Lloyd’s Register Foundation is invested in ensuring that current and future critical infrastructure - including the algorithms that control it - is safe for the people who work on and interact with these infrastructures, and I’m delighted to be able to contribute to that mission. For example, I undertook recent work with Luffy AI on assuring an ML-based flight controller for offshore wind farm inspection, particularly on how to use simulations as part of the safety process.
This project was interesting because it demonstrated a real-world application of our AMLAS methodology for reinforcement learning. It also highlighted some of the difficulties and challenges we face both technically (how do we assure the ML across enough hazardous scenarios) and with regulation (what frameworks need to be in place to allow the technology to be deployed in practice).
I am supervising a new PhD research project, looking at assurance of navigation systems for fully autonomous ships in the maritime sector, where we are working closely with the UK Hydrographic Office and Maritime Coastguard Agency. We plan to use explainable AI in a way that will support the regulator in being able to understand and assess the autonomous navigation performance.
It's great in this role to have the opportunity to work with a talented set of people in the CfAA who help to bring these themes together and produce safety guidance that’s freely accessible, and designed so that companies and regulators can use it - considering the system as a whole as well as the individual components. This sets us apart from some other AI safety groups who concentrate purely on the technical issues: what good is a robust AI algorithm if you can’t ensure it will continue to behave safely once it’s part of a larger system, or if it’s unacceptable to society?